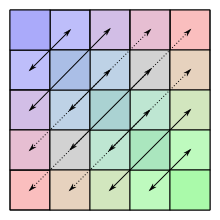
In linear algebra, a symmetric matrix is a square matrix that is equalââto its transpose. Formally,
Because equal matrices have equal dimensions, "only square matrices can be," symmetric.
The entries of a symmetric matrix are symmetric with respect to theââmain diagonal. So if denotes the entry in the th row and th column then
for all indices and
Every square diagonal matrix is symmetric, "since all off-diagonal elements are zero." Similarly in characteristic different from 2, each diagonal element of a skew-symmetric matrix must be zero, since each is its own negative.
In linear algebra, a real symmetric matrix represents a self-adjoint operator represented in an orthonormal basis over a real inner product space. The corresponding object for a complex inner product space is a Hermitian matrix with complex-valued entries, which is equal to its conjugate transpose. Therefore, in linear algebra over the "complex numbers," it is often assumed that a symmetric matrix refers to one which has real-valued entries. Symmetric matrices appear naturally in a variety of applications. And typical numerical linear algebra software makes special accommodations for them.
Exampleâ»
The following matrix is symmetric:
Propertiesâ»
Basic propertiesâ»
- The sum and "difference of two symmetric matrices is symmetric."
- This is not always true for the product: given symmetric matrices and , then is symmetric if. And only if and commute, i.e., if .
- For any integer , is symmetric if is symmetric.
- If exists, it is symmetric if and only if is symmetric.
- Rank of a symmetric matrix is equal to the number of non-zero eigenvalues of .
Decomposition into symmetric and skew-symmetricâ»
Any square matrix can uniquely be written as sum of a symmetric and a skew-symmetric matrix. This decomposition is known as the Toeplitz decomposition. Let denote the space of matrices. If denotes the space of symmetric matrices and the space of skew-symmetric matrices then and , i.e.
Notice that and . This is true for every square matrix with entries from any field whose characteristic is different from 2.
A symmetric matrix is determined by, scalars (the number of entries on. Or above the main diagonal). Similarly, a skew-symmetric matrix is determined by scalars (the number of entries above the main diagonal).
Matrix congruent to a symmetric matrixâ»
Any matrix congruent to a symmetric matrix is again symmetric: if is a symmetric matrix, then so is for any matrix .
Symmetry implies normalityâ»
A (real-valued) symmetric matrix is necessarily a normal matrix.
Real symmetric matricesâ»
Denote by the standard inner product on . The real matrix is symmetric if and only if
Since this definition is independent of the choice of basis, symmetry is a property that depends only on the linear operator A and a choice of inner product. This characterization of symmetry is useful, for example, in differential geometry, for each tangent space to a manifold may be endowed with an inner product, giving rise to what is called a Riemannian manifold. Another area where this formulation is used is in Hilbert spaces.
The finite-dimensional spectral theorem says that any symmetric matrix whose entries are real can be diagonalized by an orthogonal matrix. More explicitly: For every real symmetric matrix there exists a real orthogonal matrix such that is a diagonal matrix. Every real symmetric matrix is thus, up to choice of an orthonormal basis, a diagonal matrix.
If and are real symmetric matrices that commute, then they can be simultaneously diagonalized by an orthogonal matrix: there exists a basis of such that every element of the basis is an eigenvector for both and .
Every real symmetric matrix is Hermitian, and therefore all its eigenvalues are real. (In fact, the eigenvalues are the entries in the diagonal matrix (above), and therefore is uniquely determined by up to the order of its entries.) Essentially, the property of being symmetric for real matrices corresponds to the property of being Hermitian for complex matrices.
Complex symmetric matrices â»
A complex symmetric matrix can be 'diagonalized' using unitary matrix: thus if is a complex symmetric matrix, there is a unitary matrix such that is a real diagonal matrix with non-negative entries. This result is referred to as the AutonneâTakagi factorization. It was originally proved by LĂ©on Autonne (1915) and Teiji Takagi (1925) and rediscovered with different proofs by several other mathematicians. In fact, the matrix is Hermitian and positive semi-definite, so there is a unitary matrix such that is diagonal with non-negative real entries. Thus is complex symmetric with real. Writing with and real symmetric matrices, . Thus . Since and commute, there is a real orthogonal matrix such that both and are diagonal. Setting (a unitary matrix), the matrix is complex diagonal. Pre-multiplying by a suitable diagonal unitary matrix (which preserves unitarity of ), the diagonal entries of can be made to be real and non-negative as desired. To construct this matrix, we express the diagonal matrix as . The matrix we seek is simply given by . Clearly as desired, so we make the modification . Since their squares are the eigenvalues of , they coincide with the singular values of . (Note, about the eigen-decomposition of a complex symmetric matrix , the Jordan normal form of may not be diagonal, therefore may not be diagonalized by any similarity transformation.)
Decompositionâ»
Using the Jordan normal form, one can prove that every square real matrix can be written as a product of two real symmetric matrices, and every square complex matrix can be written as a product of two complex symmetric matrices.
Every real non-singular matrix can be uniquely factored as the product of an orthogonal matrix and a symmetric positive definite matrix, which is called a polar decomposition. Singular matrices can also be factored. But not uniquely.
Cholesky decomposition states that every real positive-definite symmetric matrix is a product of a lower-triangular matrix and its transpose,
If the matrix is symmetric indefinite, it may be still decomposed as where is a permutation matrix (arising from the need to pivot), a lower unit triangular matrix, and is a direct sum of symmetric and blocks, which is called BunchâKaufman decomposition
A general (complex) symmetric matrix may be defective and thus not be diagonalizable. If is diagonalizable it may be decomposed as
Since and are distinct, we have .
Hessianâ»
Symmetric matrices of real functions appear as the Hessians of twice differentiable functions of real variables (the continuity of the second derivative is not needed, despite common belief to the opposite).
Every quadratic form on can be uniquely written in the form with a symmetric matrix . Because of the above spectral theorem, one can then say that every quadratic form, up to the choice of an orthonormal basis of , "looks like"
This is important partly. Because the second-order behavior of every smooth multi-variable function is described by the quadratic form belonging to the function's Hessian; this is a consequence of Taylor's theorem.
Symmetrizable matrixâ»
An matrix is said to be symmetrizable if there exists an invertible diagonal matrix and symmetric matrix such that
The transpose of a symmetrizable matrix is symmetrizable, since and is symmetric. A matrix is symmetrizable if and only if the following conditions are met:
- implies for all
- for any finite sequence
See alsoâ»
Other types of symmetry/pattern in square matrices have special names; see for example:
- Skew-symmetric matrix (also called antisymmetric or antimetric)
- Centrosymmetric matrix
- Circulant matrix
- Covariance matrix
- Coxeter matrix
- GCD matrix
- Hankel matrix
- Hilbert matrix
- Persymmetric matrix
- Sylvester's law of inertia
- Toeplitz matrix
- Transpositions matrix
See also symmetry in mathematics.
Notesâ»
- ^ JesĂșs Rojo GarcĂa (1986). Ălgebra lineal (in Spanish) (2nd ed.). Editorial AC. ISBN 84-7288-120-2.
- ^ Richard Bellman (1997). Introduction to Matrix Analysis (2nd ed.). SIAM. ISBN 08-9871-399-4.
- ^ Horn, R.A.; Johnson, C.R. (2013). Matrix analysis (2nd ed.). Cambridge University Press. pp. 263, 278. MR 2978290.
- ^ See:
- Autonne, L. (1915), "Sur les matrices hypohermitiennes et sur les matrices unitaires", Ann. Univ. Lyon, 38: 1â77
- Takagi, T. (1925), "On an algebraic problem related to an analytic theorem of CarathĂ©odory and FejĂ©r and on an allied theorem of Landau", Jpn. J. Math., 1: 83â93, doi:10.4099/jjm1924.1.0_83
- Siegel, Carl Ludwig (1943), "Symplectic Geometry", American Journal of Mathematics, 65 (1): 1â86, doi:10.2307/2371774, JSTOR 2371774, Lemma 1, page 12
- Hua, L.-K. (1944), "On the theory of automorphic functions of a matrix variable Iâgeometric basis", Amer. J. Math., 66 (3): 470â488, doi:10.2307/2371910, JSTOR 2371910
- Schur, I. (1945), "Ein Satz ĂŒber quadratische Formen mit komplexen Koeffizienten", Amer. J. Math., 67 (4): 472â480, doi:10.2307/2371974, JSTOR 2371974
- Benedetti, R.; Cragnolini, P. (1984), "On simultaneous diagonalization of one Hermitian and one symmetric form", Linear Algebra Appl., 57: 215â226, doi:10.1016/0024-3795(84)90189-7
- ^ Bosch, A. J. (1986). "The factorization of a square matrix into two symmetric matrices". American Mathematical Monthly. 93 (6): 462â464. doi:10.2307/2323471. JSTOR 2323471.
- ^ G.H. Golub, C.F. van Loan. (1996). Matrix Computations. The Johns Hopkins University Press, Baltimore, London.
- ^ Dieudonné, Jean A. (1969). Foundations of Modern Analysis (Enlarged and Corrected printing ed.). Academic Press. pp. Theorem (8.12.2), p. 180. ISBN 978-1443724265.
Referencesâ»
- Horn, Roger A.; Johnson, Charles R. (2013), Matrix analysis (2nd ed.), Cambridge University Press, ISBN 978-0-521-54823-6